Learning from the past
Change in education is the norm. Reforms to national education systems are widely reported across the globe, some pertinent examples include changes from dual pathways of grammar and vocational schools to a comprehensive school model or, the transition from distributed authority for education to centralised control of curriculum and assessment and vice versa. Changes in education systems are typically associated with developments in assessment. Relatively recent examples have included a transition from end of course exams to modular approaches or, approaches to assessment beyond paper and pencil tests, instead drawing more deeply on teacher judgements using multiple approaches to collecting evidence of learning.
Technology has increasingly featured in education reform. From the introduction of computers into schools and the development curricula to raise awareness and capability in their utilisation, through to the introduction of the internet and the opportunities for engaging with knowledge and for communication this afforded. Curricula has developed from building awareness and knowledge to promoting application and transferrable skills along with moving from a distinct subject to integration across subject domains. However, assessment of Information and Communication Technology (ICT) along with Computing continues to be assessed in a traditional manner.
Even with the proliferation of devices, applications and the vanguard of new technologies in school, such VR, the process of schooling, teaching and assessment is not recognisably different. It is evident that students access knowledge in more engaging ways and convey what they know and think in a more animated means, but the process of learning and teaching have not been transformed in the manner predicted. Online schooling has emerged but is marginal, the role of the teacher remains central and millions of students across the world will undertake paper and pencil examinations across the world in the coming weeks and months. This seems at odds with the manner in which technology has transformed nearly every other part of life and work.
What can we learn from the points above?
- Reform to national education systems are complex and slow, even with regards to technology.
- Technology has broadened the way students access and present knowledge along with how they communicate.
- Schools and the process of teaching and assessment has not been transformed, despite the introduction of devices, internet and a wide array for applications.
- Formal assessment practices remain unchanged or when technology is utilised, the focus remains on assessing prescribed knowledge and its application in known contexts.
In conclusion, education systems are constantly evolving and reforming. The introduction of technology has added a new dimension to education but much of the process of teaching, learning and assessment remains comparable with previous approaches. The reasons for this, it is argued, is that hitherto, technology has focused on enhancing access to and exchange of knowledge. Learning is complex and how students are exposed to knowledge is only one part of the process. Furthermore, the role of technology in assessment has, in the main, provided efficiency in assessment of knowledge within traditional curriculum domains.
Global social and economic conditions are key drivers for education reform and there is fervent support from many stakeholders. But how is technology to be more deeply integrated into the new landscape of education and what are the implications for assessment?
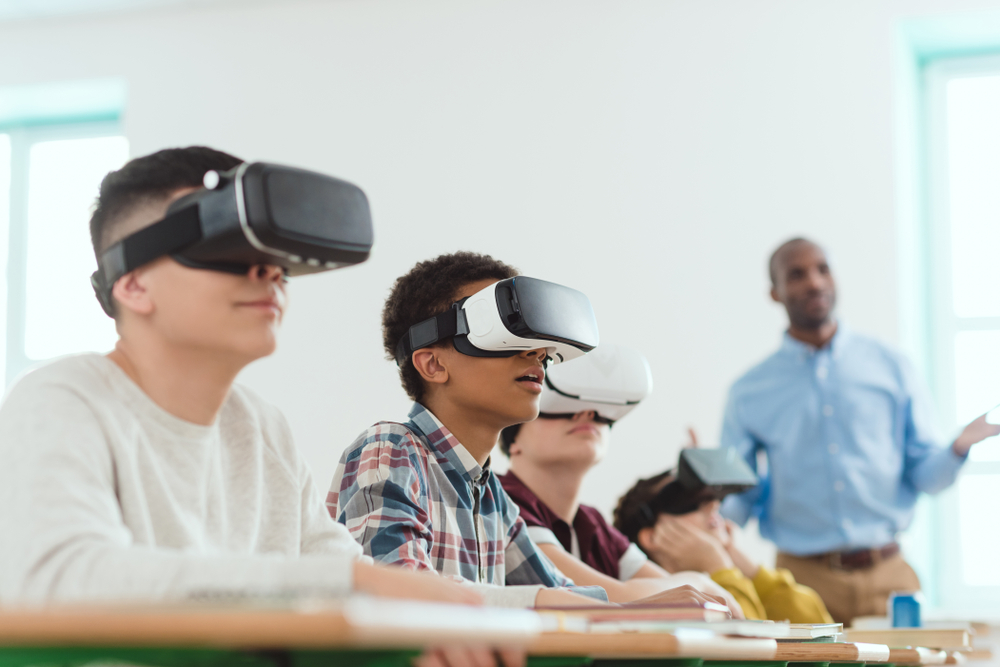
Looking to the future
Very recent developments in technology are becoming transformative for the way we live and work. But will this be equally profound for schools and the process of education? Probably. It is postulated that the impact will be defined by aspirations for how technology is to be utilised.
Technology is now able to operate in a manner hitherto impossible. Not only can it provide access to knowledge, but it can generate it. Information can be analysed, synthesised and even evaluated in a way that generates broadly accurate products that we can utilise. This step forward, it is argued here, is how technology can get at the heart of what happens in schools and learning. The capacity for technology to learn, predict and operate ‘intelligently’ is now pervasive in very many sectors and is emerging in education, artificial intelligence (AI) is unequivocally upon us. It is vital that schools embrace it, make students aware of the possibilities and risks of it and model how it’s power can be effectively harnessed. There remains, however, a need for more systematic teacher training and appropriately developed curricula.
Generative AI is already being utilised by educators for effective preparation of teaching materials. For example, assessment items can be generated that are differentiated for demand, style of question or scaffolded to support the reading proficiency of students. The reality however, this is increased efficiency and not deeply impacting on how we teach and importantly, approaches to assessment.
Technology and assessment
The role of assessment in learning has been deeply researched. In this article, a simple definition of assessment will be used: the process of gathering evidence on what learners know and can do. It is important to appreciate there exists a gap between what actually exists in terms of mental construct in the mind of a learner and the evidence gathered through the process of evidence collection as a means of determining the nature of what students know and can do. In essence, the process of sampling evidence through assessment activities renders information that is rarely entirely accurate. Therefore, all assessment practices are prone to errors. But highly effective educators are able to design and implement assessment activities that reduce errors in the evidence and are able to take account for them when making judgments about what a student knows and can do.
The complexity of assessment means that the standard assessment paradigm (SAP) described by Swiecki et al. (2024) results in teachers generating often used assessment tasks that are:
- Onerous to produce
- Not appropriately reflective of purpose
- By design, lack validity and reliability
- Discrete and not reflective of progress
- Lack personalisation
Swiecki et al. (2024) and others argue that this serves as an opportunity for AI to assist with assessment. In a review of the application of AI to assessment the following is noted:
- Application of AI to simplistic assessment methods such as multiple-choice questions is widely used but deeper analysis reveals significant investment is needed to reproduce basic levels of teacher produced assessments and existing AI models do not always display high levels of reliability
- The use of AI to assist in improving reliability in peer assessment is reported with varying degrees of success
- AI is demonstrating some effect in writing analytics for extended piece of work
- Electronic assessment platforms and gamification applications yield improving evidence of assessment knowledge and its application. When adopting multimodal approaches to data collection, AI derived methods can provide salient evidence of the progress in learning.
- Computerised Adaptive Test (CATs) can provide greater levels of precision
- Digital simulated tasks can provide learners opportunities to apply learning in known and unfamiliar contexts with reported effectiveness
- Ultimately, one of the benefits of AI and technology in assessment is that it permits the learner to externalise knowledge in multimodal ways which enhances that act of assessment
This list provides an introduction to the ways AI and its applications are beginning to be used in assessments. However, there remains some distance from AI becoming a powerful driver for assessment. Researchers in the field list following areas for consideration when working with AI models of assessment: (i) existing applications require the expertise of the teacher to draw on AI generated data critically and only as a reference, (ii) current AI approaches are not yet effectively linked to pedagogical and formative utilisation of assessment, (iii) assessment practices using AI have a narrow focus on what is learned and ultimately, (iv) as AI systems for assessment and improve, the possibility of having ongoing assessment of students creates concerns about control and compliance. Mermian and Doleck (2024) suggest that one of the limitations of AI systems is that it not always appropriately aligned with learning theories. Consequently, it is argued AI driven assessments are limited to supporting teachers with (i) interpretation responses to recall of knowledge, (ii) assisting with translation, (iii) text generation in different presentation forms and (iv) analysis and summary of data.
A pertinent point raised above relates to the relative narrow approach to assessment of what students know and can do by AI systems. Memarian and Doleck (2024) support this claim in their work on AI and it relationship with assessment for learning (AfL). A definition for AfL is the process of teachers establishing criteria of successful learning of a specific learning objective, gathering of evidence of student performance against the criteria, using the evidence formatively to provide feedback to the teacher and learner to scaffold progress towards meeting the learning objective. There is a body of evidence that indicates AfL is highly effective in supporting learning (Wiliam et al., 2024).
A review of the research literature of the use of AI for AfL reveals that there are relative few studies drawing an quantitative evidence to demonstrate impact (a single article) with most work focusing on qualitative analysis of student perceptions (Memarian and Doleck, 2024). Most AI activity is focused on assessment of learning. DiCerbo (2020) suggests that teacher pedagogy and AfL is sophisticated and subtle in nature when applied to a diverse range of students whilst, AI is not yet able to exhibit the same level of operation for informing classroom instruction, accounting for differences in sthe starting point and progress of learners and for real life applications. Nevertheless, the variability in the successful impact of AfL (Wiliam, 2018) offers an opportunity for AI to make a central contribution of the effective implementation of assessment as a driver for learning. The proposals outlined by Memarian and Doleck (2024) suggest a pertinent way forward on this issue.
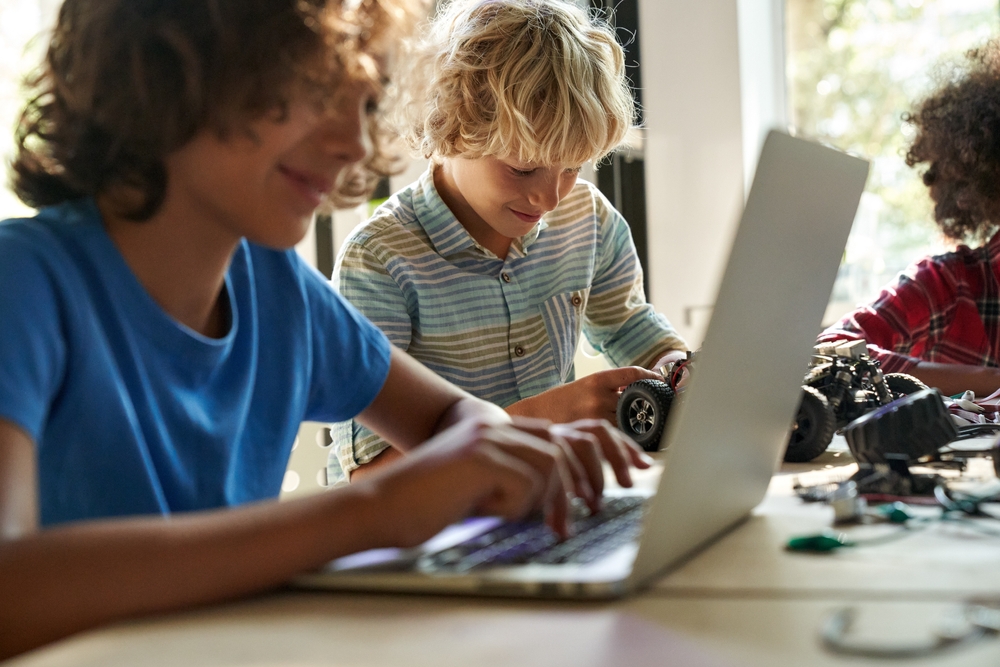
Possibilities for technology and assessment
Trends in education reform differ to that raised in the first section of this article. The desired product from education is viewed through a different social and economic paradigm, as such there is an ambitious definition for student wellbeing, ways of thinking, transferrable skills and the environment that have largely played a peripheral role hitherto. The product from education is to be young adults with the capacity to manage their own wellbeing, to possess values and character, skills and knowledge that allow them be effective citizens, to display effective interpersonal, communication and linguistic skills, to be digitally literate and socially committed.
To achieve these aspirations for education reform will require changes to curriculum structure, pedagogical approaches and fundamentally, assessment methodology. With reference to the latter point, there are implications for not how we assess but what is assessed. There is a need to effectively assess:
- Student wellbeing
- Ways of knowledge application and thinking, for instance criticality or creativity
- Self-regulatory and learning capabilities
- Communication, linguistic and interpersonal skills
- Digital literacy
Such features of learning are unlikely to ever be assessed through traditional paper and pencil tests. Assessment of proficiency level for managing self-regulation, creativity or effective interpersonal skills will rely on alternative evidence sources, perhaps observations of student behaviours, actions and decision making. This is the realm of technology, this is when technology can transition from being an effective provider of knowledge to assist the learning to the means by which data is generated on learning and development. Machine learning is making these aims possible. Existing frameworks for assessing student wellbeing, engagement or cognitive challenge in tasks exist and through the use of technology, these frameworks can be applied to gather data to inform the teacher instantaneously how students are feeling, how committed they are to learning and whether the activity is too demanding. It can produce standardised assessments of features of learning that extend beyond traditional assessment practices.
The opening section identified the global social and economic conditions as drivers for education reform and asked how technology to become more deeply integrated into the new landscape of education? The opportunities presented above, it is argued here, is a way forward to achieving it.
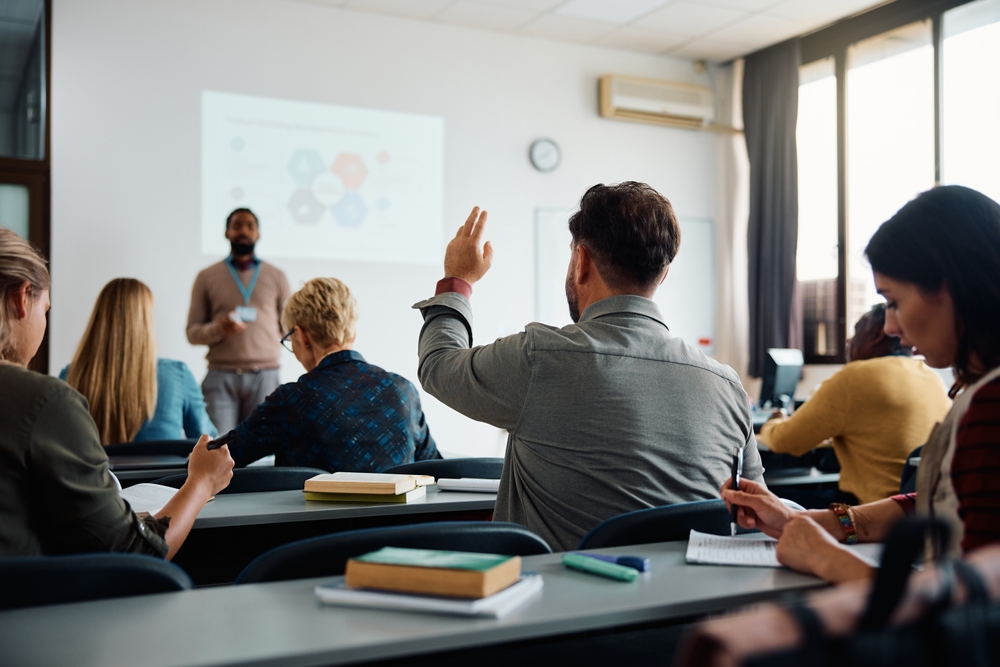
Next steps
Education systems are not yet in a position to implement the possibilities described in the previous section, but they will emerge if desired. Technology alone is not the determining factor for realising these aims. The following are proposed areas for development to deliver on education reforms:
- Teacher training
Whilst initially there is a requirement to upskill teachers in the utilisation of emerging technologies, the greater challenge is to restructure initial teacher education and continuing professional development to focus on teaching and assessing for student wellbeing, application of knowledge in a creative or critical manner, developing learning and self-regulatory skills, promoting interpersonal and communication skills and integrating digital literacy. This will need to occur within the fabric of recognisable curriculum domains and through integrated projects.
- Curriculum
Education systems will need to considerably revise curriculum such that explicit teaching of wellbeing, ways of knowledge application and thinking, for instance criticality or creativity, self-regulatory and learning capabilities, communication, linguistic and interpersonal skills, along with digital literacy. These reforms will need to be accompanied by associated guidance and training.
- Research
The content above indicates that more refinement is needed in the application of AI in assessment but that the opportunities are for advancing education are plentiful. Integration of empirical research conducted in universities in collaboration with school led application of research may serve as an effective means of accelerating implementation of reforms.
- Trusted and recognised AI assessment methodology
Only with the points above addressed can trusted AI assessment methodology display the validity and reliability so that they are trusted as a means of providing evidence of learning alongside the existing SAP.
However, the desired changes to education are driven by social and economic imperatives that cannot wait for the hitherto inconsistent nature of education reforms reported for national systems. Perhaps a way forward is to draw on a collaboration between higher education, private international schools and the national education systems. International schools operate with greater flexibility and display greater dynamism in education development than national systems. Furthermore, they often have greater resource and opportunity to implement new technologies. Learning from the context of international schools can be exchanged with national systems to enhance progress.
Discover innovative solutions, trusted providers, and forward-thinking resources designed to support schools, educators, and learners—on AISL Mall.
References and Further Reading
- DiCerbo, K. (2020) Assessment for learning with diverse learners in a digital world. Educational Management: Issues and Practice, 29(3), 90-93.
- Laevers, F & Declercq, B (2018). How well‐being and involvement fit into the commitment to children’s rights. European Journal of Education, 53, 325–335.
- Memarian, B and Doleck, T. (2024) A review of assessment for learning with artificial intelligence. Computers in Human Behaviour: Artificial Humans (2).
- Swiecki, Z. et al., (2022) Assessment in the age of artificial intelligence. Computers in Human Behaviour: Artificial Humans (3).
- Unseco (2024), AI and Education: Guidance for Policy Makers.
- Wiliam, D. (2018) Assessment for Learning: Meeting the challenge of implementation.
- Wiliam, D., Lee, C., Harrison, C. & Black, P. (2004) Teachers developing assessment for learning: Impact on student achievement. Assessment in Education: Principles, Policy & Practice, 11(1), 4-65.